Artificial Intelligence Is Shaping the Future of the Pharmaceutical Industry
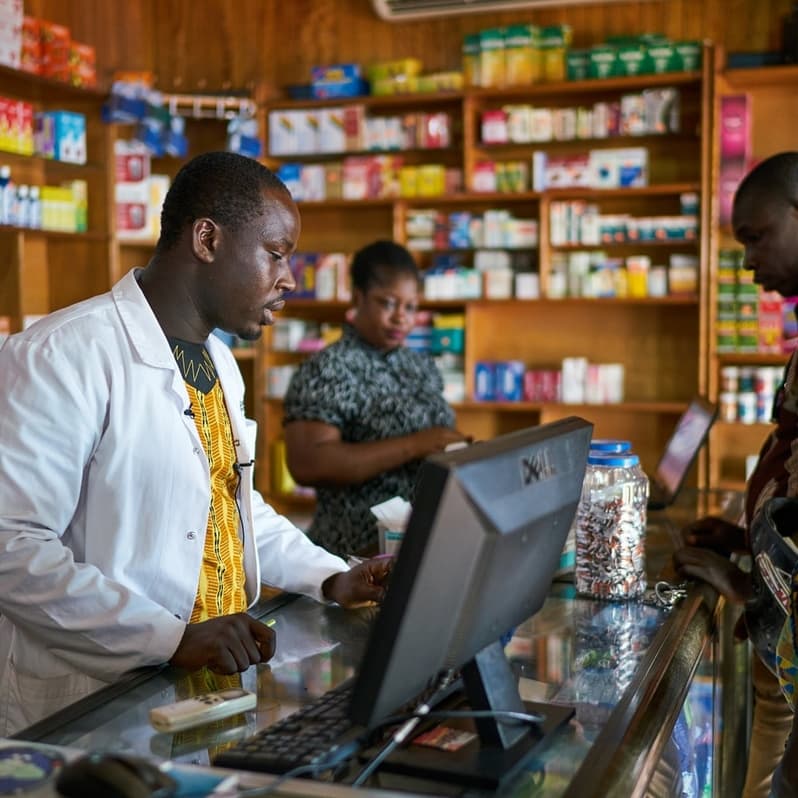
Pharmaceutical companies have long made use of cutting-edge technologies to ensure the safe and reliable delivery of their products to market. The ongoing COVID-19 pandemic has shown us how important it is to get medications and vaccines to market as quickly as possible.
However, researching and developing a new drug takes on average ten years and costs about $1 billion. It’s also worth mentioning that only 10% of them make it past trial and regulatory approval, which is why most experts in the industry agree that finding ways to make the process more efficient is imperative.
These days, it’s virtually impossible to talk about the future of any industry without bringing up artificial intelligence (AI). AI has been met with a mix of excitement, mistrust, and fear since its inception. It’s also been frequently heralded as a game-changer for its potential to improve established business tactics and opening up new avenues for meeting and resolving important pain points in a wide range of industries.
AI integration in drug discovery and development is motivated by its ability to handle large amounts of data more efficiently. In the pharmaceutical industry, artificial intelligence refers to the use of automated algorithms to perform activities that typically required human intervention.
It’s a technology-based system that combines a variety of complex tools and networks to simulate human intelligence, although it does not threaten to completely replace human physical presence, contrary to common fears. AI employs systems and software that can interpret and learn from data. With enough training, it can make decisions on its own in order to reach predefined goals.
Machine learning (ML) is a subset of AI or a method to realize AI that involves training algorithms to recognize patterns in data and make autonomous decisions. AI also includes other methods such as knowledge representation and solution search.
Deep learning (DL) is a subfield of machine learning based on artificial neural networks (ANNs) – sets of perceptrons or artificial neurons, which are essentially interconnected nodes that mimic human biological neurons and the transmission of electrical impulses between them.
ANNs can use single-linked or multi-linked algorithms to solve problems. ANNs based on either supervised or unsupervised training strategies include multilayer perceptron (MLP) networks, recurrent neural networks (RNNs), and convolutional neural networks (CNNs).
MLP networks are used in pattern recognition, process identification, optimization aids, and controls as universal pattern classifiers. RNNs are closed-loop networks that can memorize and store information. CNNs are deep neural networks that use a set of dynamic systems with local connections and have one or more convolutional layers.
Convolution refers to the process of applying a filter to the input. For example, you give the algorithm an image as input, and it will be able to distinguish between various aspects or objects of that image by giving them different values.
CNNs are most commonly used to classify images, but they can also be applied to biological system modeling and processing complex brain functions.
The field of AI is very broad, with many specializations such as perception, natural language processing (NLP), robotics, machine vision, and speech recognition, just to name a few. However, the scope of this article is not to explore them all but to discuss AI is shaping the future of pharma.
How AI Is Currently Being Used in Pharma
According to the McKinsey Global Institute, AI and machine learning in the pharmaceutical industry could generate approximately $100 billion, and that’s only in the US healthcare system. The use of AI benefits physicians, consumers, insurers, and regulators by improving decision-making, maximizing innovation, and helping the pharmaceutical industry deliver safer, more affordable treatments much faster than before.
Roche, Pfizer, Merck, AstraZeneca, GSK, Sanofi, AbbVie, Bristol-Myers Squibb, and Johnson & Johnson are among the top pharmaceutical companies that are already using AI technology.
The cost of creating new pharmacological therapies and the time it takes to bring them to market have risen. This is largely due to the complexity of the illnesses that the industry is currently focused on. Since effective treatments for common illnesses have already been found, the emphasis is now on rare diseases that involve complex chemistry.
The process of developing a new drug begins with identifying the drug’s target. Having a thorough understanding of how the molecules will interact is absolutely essential. This is something AI can help with since it can be used to compare the biochemical and biophysical properties of millions of molecules with the structures and properties of hundreds of thousands of proteins and find the molecules that have a high probability of binding to the target.
Artificial intelligence algorithms can be used to simulate over a billion interactions and narrow down the list of candidate compounds. These tests also provide additional information needed to refine the list further. This part is iterated until the algorithms can give researchers a manageable list, saving time and money in the process. AI can shorten the time it takes to develop a new pharmaceutical treatment by up to 75% and lower costs by 80%.
Because AI performs so well on predicting drug-target interactions, it can also be utilized to find new uses for existing medications, further reducing costs and time because a repurposed drug can qualify for Phase II clinical trials straight away since they have known safety profiles.
These AI drug-target interaction studies can also estimate the possibility of polypharmacology, which refers to a drug molecule’s propensity to interact with multiple receptors increasing the risk of adverse effects. By employing Bayesian classifiers and SEA algorithms to comb through massive databases and discover links between pharmacological drug profiles and possible targets, AI can aid in the development of safer medications.
In 2018, MIT pretended with Pfizer and Novartis to improve the drug design and manufacturing process through the Machine Learning for Pharmaceutical Discovery and Synthesis Consortium, which aims to identify and address the most significant issues.
AI has the potential to revolutionize drug discovery by shortening the research and development timeline, making pharmaceutical treatments more affordable, and increasing the likelihood of FDA clearance.
The recent dramatic rise in activity in the deployment of AI capabilities in the pharmaceutical industry shows no signs of abating, with over half of global healthcare companies making plans to incorporate the technology in their operations by 2025.